By optimizing data and leveraging information in real time, they had more efficient and faster decision-making. However, to capture these opportunities and manage smart material flows and sustainable ecosystems, a Production Management System (PMS) also needs an I4.0 upgrade.
Automation, increased computing power, real-time data analytics and machine learning (ML) enabled the I4.0 revolution. From these technological developments in automation technology, real-time data collection and visualization, also digital twins emerged. In the metals industry, digital twins are currently available not only for production lines but also for consistent data collections of material units along their production route. Additionally, digital twins are used for transports in the stockyards, enabling transparency and efficiency along the whole smart production process.
Such models are generated, trained and validated out of historical data of processes and products. Their added value lies in the capability of predicting future results based on new data inputs.
Smart supply chain - The next generation of supply chain
Digital steel production management will increasingly involve close collaboration between human agents like a scheduler or yard operator, and smart autonomous agents. These agents are small pieces of software that focus on a very specific set of problems. They can make independent local decisions and notify human agents or even other smart agents working together to solve larger problems by communicating together in Multi-Agent Systems (MAS). Such agents can be incorporated as part of business process workflows and can be enhanced through AI in order to detect changes in their environment, learn from the impact of decisions and adapt their behavior accordingly.
Through the real time availability of big data from the end-to-end supply chain, computational power, digital twins, and ML algorithms, we have all the ingredients to build smart supply chains. That is, supply chains that are able to take faster and better decisions. Reducing latencies in decision-making and in reacting to flow disruptions is one of the biggest potential benefits of the digitalization of supply chains. Any supply chain event will be visible to human decision-makers immediately.
Smart processes and products emerge
Single solutions have been developed in the past years to leverage the potentials of Industry 4.0 in different areas of steel production. At the melt shop, PSImetals Online Heat Scheduler (OHS) manages and optimizes the detailed work schedules for the heats. Based on dedicated work plans, it schedules all required treatment and transport steps, assigns production lines and operating equipment based on available capacity in real-time. It gives an overview of the current versus planned progress for all heats. OHS always ensures the seamless delivery of heats and sequences to the caster and reacts in case of any disruptions or breakdowns.
In quality control, predictive algorithms are used to evaluate the risk of deviation of a target feature at any stage of production. Feeding the algorithm with as-is data for the upstream process and expected values of the downstream process enables the use of the algorithm at different intermediate production stages. In case of out of range prediction, a prescriptive algorithm would be called to suggest for each material unit the best possible downstream parameters, in order to react to the identified deviation risk and nudge the material to the possible best result according to the available knowledge.
In addition to processes, steel and aluminium products themselves are becoming smart. PSI Metals’ partner coilDNA developed a technology that gives steel and aluminium pieces an identity. The technology turns them into intelligent products by allowing you to track their history.
Scaling-up smart materials and process flows
To capture opportunities and manage smart material & process flows, a Production Management System need an Industry 4.0 upgrade. There are some building blocks required for such an upgrade.
The first step would be to break the traditional automation levels pyramid as shown below.
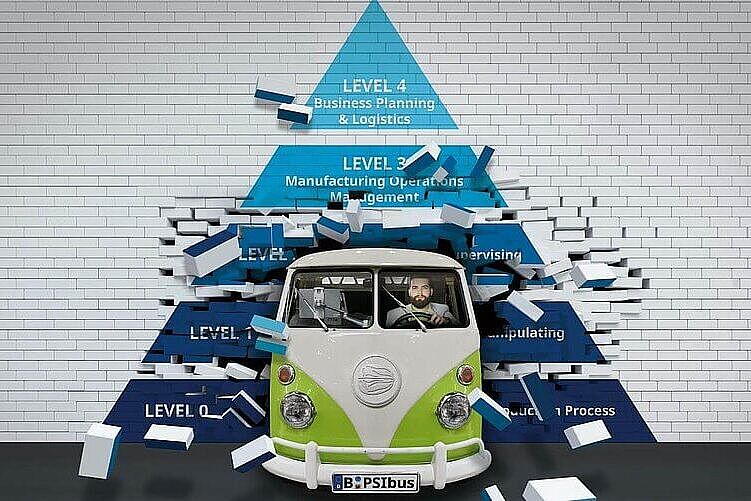
This structure has indeed two main limitations:
- Communication: Generally, one level can only communicate with the levels directly above or below it
- Data abstraction and/or translation: This occurs when passing from one layer to another, limiting data to certain layers and not to others. This makes it difficult to integrate or connect a ML service that would require data from different levels.
The next, would be for a Production Management 4.0 System to provide an open platform architecture that enables a flexible orchestration of business process flows. More specifically, a dedicated workflow management service should support configuring customized and adaptive business processes. This may involve native functions from different business areas such as quality control or production planning, but could also integrate external systems and services such as an ML-based defect prediction service.
In addition to core Advanced Production Scheduling and Manufacturing Execution System services, a Production Management 4.0 System needs to provide state-of-the-art embedded business intelligence, User Interface configuration, authentication, authorization, and event notification services. One such system is the PSImetals Service Platform.
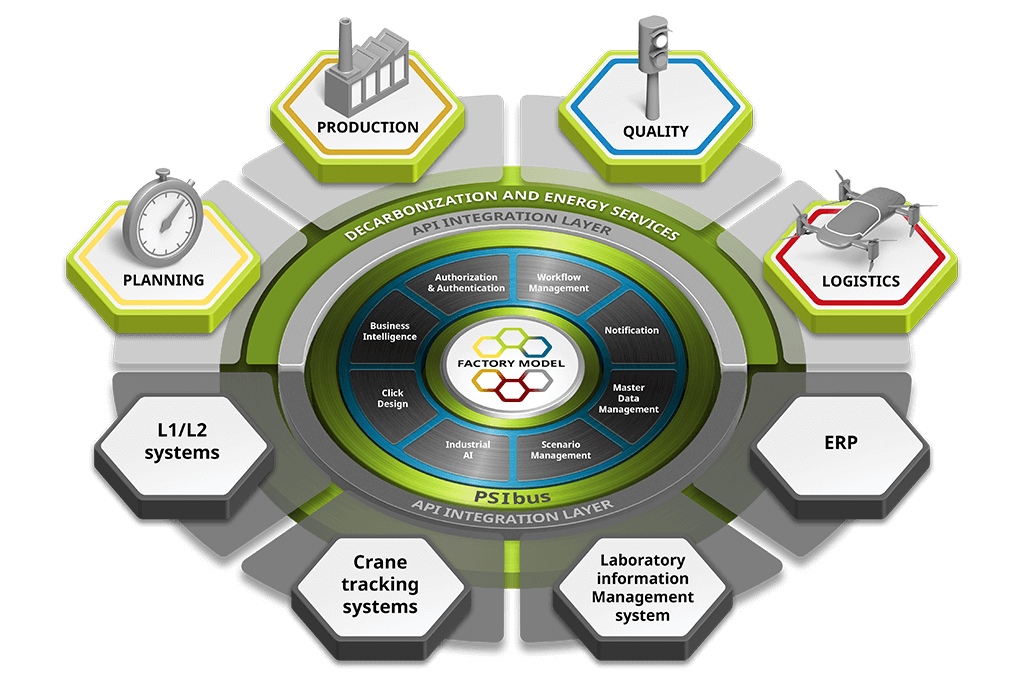
PSImetals PMS enables integrated services
As an example of how different digital services can be integrated by a modern PMS, let us look at the case of hot rolling schedule adaptation. A machine learning model might detect quality non-conformities involving one or more scheduled slabs. A dedicated planning service will de-schedule the slab(s) and check the feasibility and quality of the remaining rolling sequence. Depending on the answer, the check flow might end or continue with the search for alternative slab candidates.
In an e-commerce platform, many functions of the PMS could be called as a service. Using the buyer’s data as input, a dynamic Order Dressing, rule-based, could answer feasibility inquiries, including first-time inquiries. The Due Date Quoting planning function could then use the results of the production order elaborated by Order Dressing to estimate the delivery date. And after the purchase, all material data could be retrieved by calling the Quality Management service. For this, the e-commerce platform needs to be securely connected to the PM services, via PSIbus.
Decarbonization?
In a KPI-driven PMS, it might be possible to balance classical KPIs like quality, throughput, stock or due date with new KPIs like efficient energy consumption and reduced CO2 emissions. This way, not only the best compromise could be identified, but the impact on sustainability of any decision would get transparent, enabling sustainable steel production.
It is clear that the ongoing digital transformation promises to make steel material and production workflows smarter and greener. We anticipate more improvements in the planning and production process. As AI take more repetitive and data-driven tasks, the role of human agents will switch to focus on tasks that AI are not yet capable of performing like creative thinking, innovation, scenario assessment and people management.