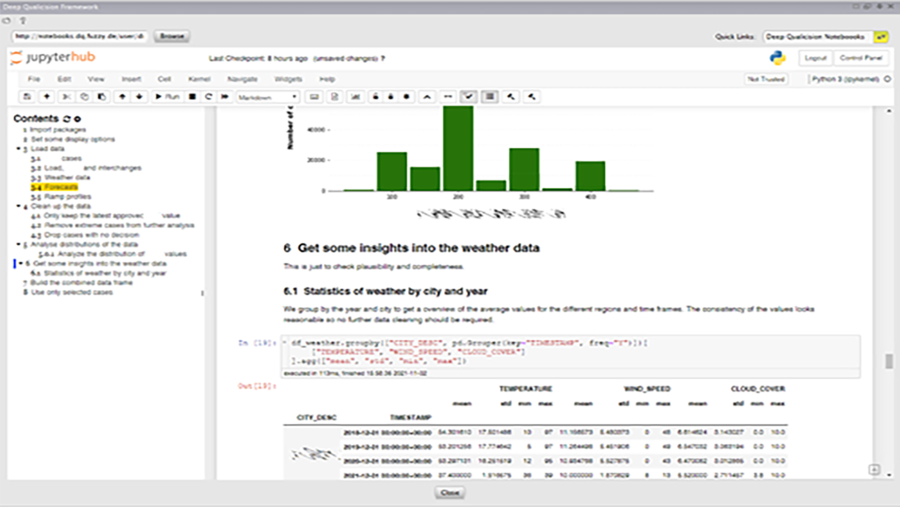
The PSIdeepqualicision AI Framework efficiently learns how to set the parameters of decision and optimization algorithms (DOA) so that almost any DOA procedure working on business process data can automatically adjust itself. At its heart is a machine learning method based on the automatic detection of KPI goal conflicts in the process data of business processes using Extended Fuzzy Logic.
Benefits at a Glance
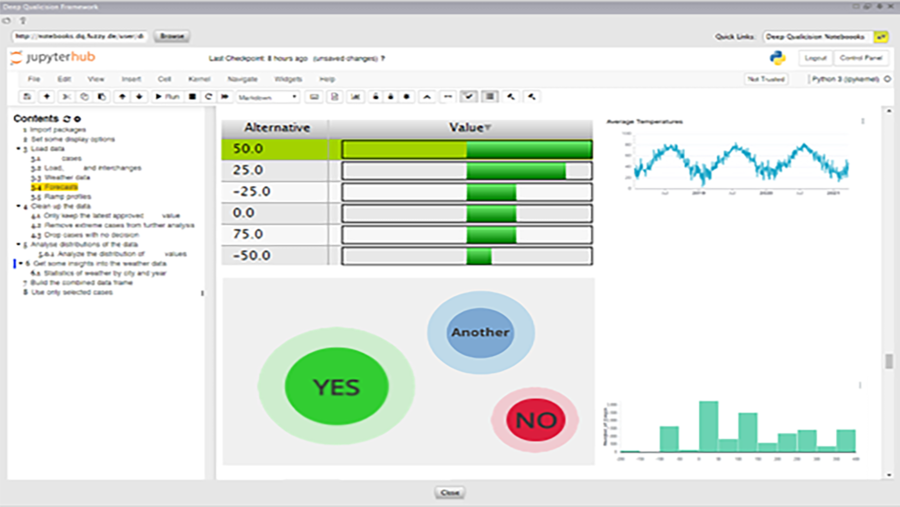
-
Self-learning data preparation Advanced machine learning methods and in-depth forecast-oriented analyzes.
-
Machine Learning Machine learning based on qualitative labeling with decision and optimization algorithms.
-
Powerful Decision-making Direct access to the powerful Qualicision AI decision-making core.
-
Modern AI stack Availability of all modern algorithms and AI methods for machine learning in Python.
-
AI Predictors Generic prediction algorithms using Qualicision AI predictors.
-
Tailored User Concept User concept according to different user groups (operator, administrator, data analyst).
-
Integrated Didactics Comprehensible application possibilities and playful interaction through integrated dialog guidance and exercise examples (Integrated Didactics).
-
Web and cloud-enabled Installation and access via PSI Marketplace and PSI cloud at the touch of a button.
-
Explainable AI results Explainable relationships amongst other things of profitability and sustainability KPIs.
-
User acceptance Increasing user acceptance due to comprehensible AI decisions.
-
Independency Process data analyses and forecasts across all contexts and industries.
Features
-
Qualitative Labelling Qualitative Labelling as part of the Qualicision AI framework optimizes raw business process data for machine learning applications by qualitatively assessing measurable data directly from business processes in the context of KPIs (Key Performance Indicators) and analyzing relationships based on this. In this way, an algorithmic bridge is automatically created between the unprocessed raw business process data and artificial intelligence (AI) methods, which significantly simplifies the time-consuming process of manual data analysis for labeling data.
-
Qualicision Impact Matrix The input for the software essentially consists of two main components: Firstly, data streams of the business process to be analyzed are recorded and automatically converted into time series with the help of time stamps. Secondly, key performance indicators (KPIs) and their value ranges, which are to be used to analyze the business process in question, are agreed with the process owner. In addition, the value ranges of the KPIs are divided into preferred and non-preferred value ranges. Green labels stand for preferred or good signal areas, while red labels connote areas that are critical, i.e. not desirable from a process perspective.
-
Learning algorithm F9118 For example, the F9118 learning algorithm enables the automatic calculation of consistent solution and decision alternatives that combine historicized data contexts with current data situations. The decision options are prepared in such a way that the user acts like a Java-based PSI click designer and operates in the PSIdeepqualicision AI Framework by clicking and navigating in selection menus and other graphically prepared GUI elements.
Modules
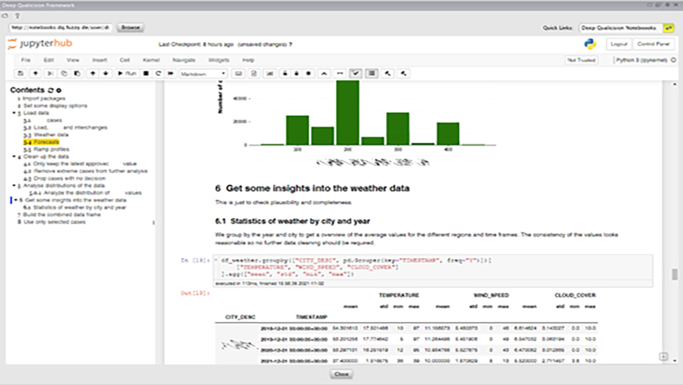
Data Analyst
Full access to the PSIdeepqualicision AI stack and all AI functions via corresponding Python imports and Jupyter notebooks.
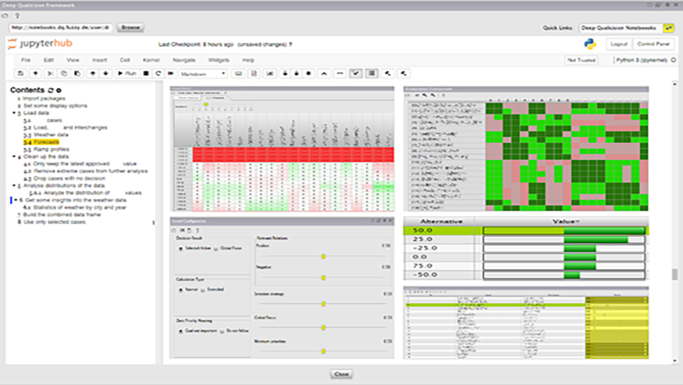
Administrator
Configuration and parameterization of the application as well as its modification and extension at the level of process KPIs.
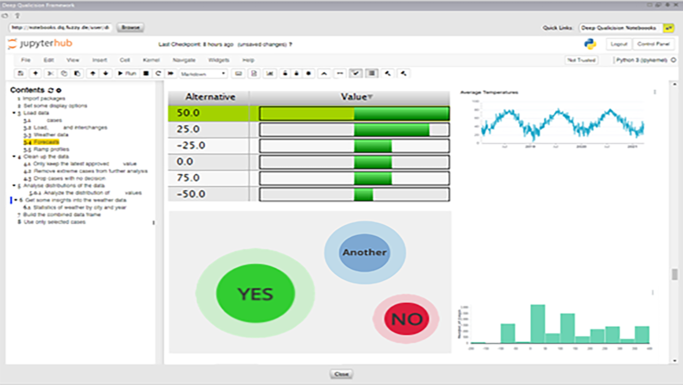
Operator
Process control and time series feedback information for the rolling training of a self-learning AI.
Typical Users
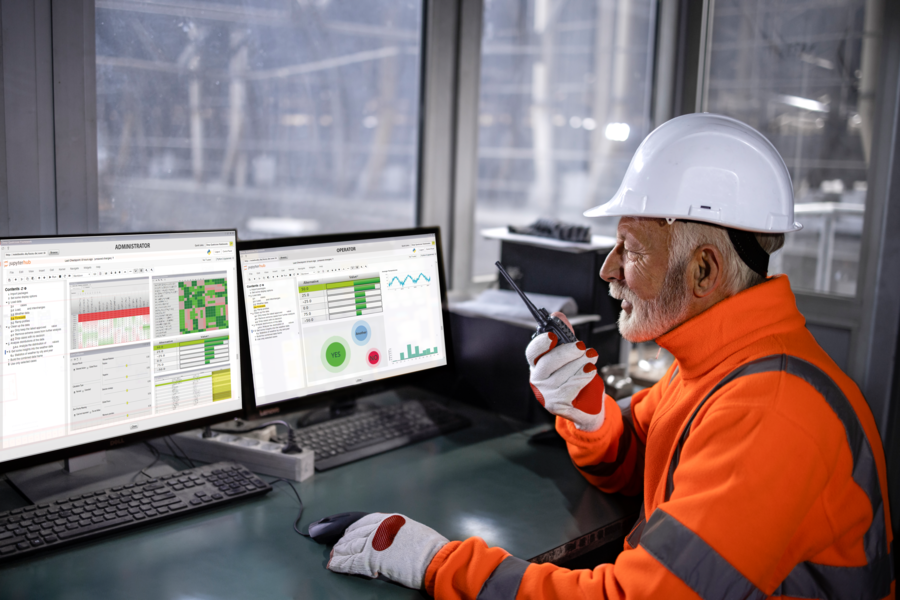
Operator
A smooth and efficient production process is the be-all and end-all for the traditional user of decision support and optimization software. Intuitive access to all relevant functions is just as important to them as the confidence that all inputs and calls are reliably processed by the system and that the software results are comprehensible and explainable.
Preconfigured systems provide KPI-oriented, comprehensible recommendations for action for users who rely exclusively on process knowledge and who can incorporate the results into their business processes and continue to use them there. This user group acts accordingly in the sense of system operators.
-
Explainable AI results
Explainable relationships amongst other things of profitability and sustainability KPIs.
-
Optimized decision making
Optimization and decision-making for selection and classification tasks.
-
Integrated Didactics
Comprehensible application possibilities and playful interaction through integrated dialog guidance and exercise examples (Integrated Didactics).